Full Professor of Ecology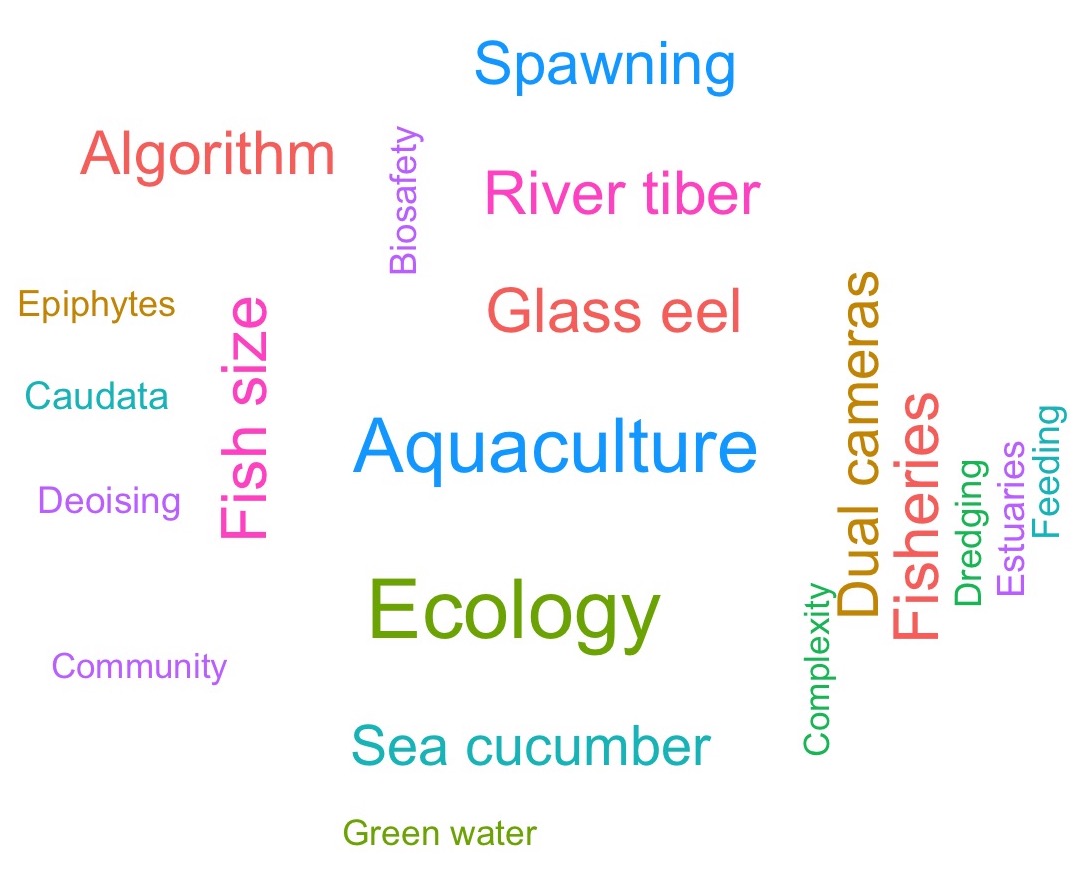
Department of Biology
Laboratory of Experimental Ecology and Aquaculture
Tel. 06-7259-5991
e-mail mscardi@mclink.it
Web: www.michele.scardi.name
Latest publications
- 2020 – Chemical signatures of femoral pore secretions in two syntopic but reproductively isolated species of Galápagos land iguanas (Conolophus marthae and C. subcristatus) – Scientific Reports – 10.1038/s41598-020-71176-7
- 2020 – Modelling matter and energy flows in the biosphere and human economy – Ecological Modelling – 10.1016/j.ecolmodel.2020.108984
- 2020 – Structure and environmental drivers of phytoplanktonic resting stage assemblages in the central Mediterranean Sea – Marine Ecology Progress Series – 10.3354/meps13244
- 2020 – Embedding ecological knowledge into artificial neural network training: A marine phytoplankton primary production model case study – Ecological Modelling – 10.1016/j.ecolmodel.2020.108985
- 2020 – Modeling macroalgal forest distribution at mediterranean scale: Present status, drivers of changes and insights for conservation and management – Frontiers in Marine Science – 10.3389/fmars.2020.00020
- 2020 – A Machine Learning approach to the assessment of the vulnerability of Posidonia oceanica meadows – Ecological Indicators – 10.1016/j.ecolind.2019.105744
- 2019 – A model predicting the PSP toxic dinoflagellate Alexandrium minutum occurrence in the coastal waters of the NW Adriatic Sea – Scientific Reports – 10.1038/s41598-019-40664-w
- 2019 – Rummaging through the bin: Modelling marine litter distribution using Artificial Neural Networks – Marine Pollution Bulletin – 10.1016/j.marpolbul.2019.110580
- 2019 – Predicting Fishing Footprint of Trawlers From Environmental and Fleet Data: An Application of Artificial Neural Networks – Frontiers in Marine Science – 10.3389/fmars.2019.00670
- 2019 – Quantifying the impact of linear regression model in deriving bio-optical relationships: The implications on ocean carbon estimations – Sensors (Switzerland) – 10.3390/s19133032
- 2019 – An ecologically constrained procedure for sensitivity analysis of Artificial Neural Networks and other empirical models – PLoS ONE – 10.1371/journal.pone.0211445
- 2019 – Artificial reproduction of Holothuria polii: A new candidate for aquaculture – Aquaculture – 10.1016/j.aquaculture.2018.08.060
- 2019 – Trends in effort and yield of trawl fisheries: A case study from the Mediterranean Sea – Frontiers in Marine Science – 10.3389/fmars.2019.00153
- 2018 – Cascaded neural networks improving fish species prediction accuracy: The role of the biotic information – Scientific Reports – 10.1038/s41598-018-22761-4
- 2018 – Modelling the vertical distribution of phytoplankton biomass in the mediterranean sea from satellite data: A neural network approach – Remote Sensing – 10.3390/rs10101666
- 2018 – A depth-resolved artificial neural network model of marine phytoplankton primary production – Ecological Modelling – 10.1016/j.ecolmodel.2018.05.003
- 2018 – Spawning and rearing of Holothuria tubulosa: A new candidate for aquaculture in the Mediterranean region – Aquaculture Research – 10.1111/are.13487
- 2017 – Analysis of phytoplankton assemblage structure in the Mediterranean Sea based on high-throughput sequencing of partial 18S rRNA sequences – Marine Genomics – 10.1016/j.margen.2017.06.001
- 2017 – Shoot micro-distribution patterns in the Mediterranean seagrass Posidonia oceanica – Marine Biology – 10.1007/s00227-017-3121-1
- 2017 – Bight of Benin: a Maternal Perspective of Four Beninese Populations and their Genetic Implications on the American Populations of African Ancestry – Annals of Human Genetics – 10.1111/ahg.12186
- 2016 – Dataset exploited for the development and validation of automated cyanobacteria quantification algorithm, ACQUA – Data in Brief – 10.1016/j.dib.2016.06.042
- 2016 – Modeling landings profiles of fishing vessels: An application of Self-Organizing Maps to VMS and logbook data – Fisheries Research – 10.1016/j.fishres.2016.04.005
- 2016 – ACQUA: Automated Cyanobacterial Quantification Algorithm for toxic filamentous genera using spline curves, pattern recognition and machine learning – Journal of Microbiological Methods – 10.1016/j.mimet.2016.03.007